Best Warehouse-Native Analytics Tools in 2025

Detailed comparison of the best warehouse-native analytics software
What is warehouse-native analytics?
Warehouse-native analytics accesses your data directly top of your data warehouse instead of moving it to third-party tools for processing. Traditionally, businesses have used reverse-ETL to transfer data into external analytics tools, which can be time-consuming and often slow the workflow. Warehouse-native analytics skips that step, allowing you to analyze your data inside the data warehouse.
Key benefits:
- 100% reliability, 100% ownership
- Automated SQL query generation
- Eliminate data duplication and data silos
- Cost efficiency
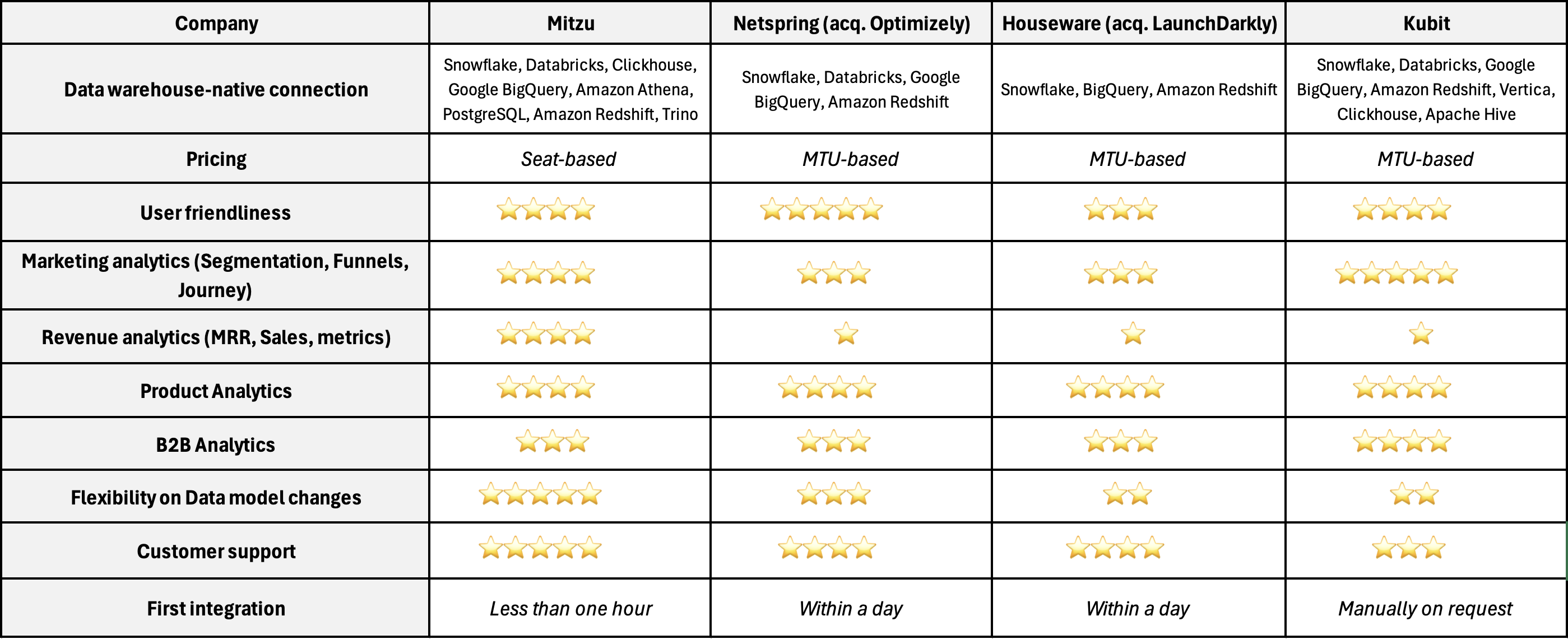
Mitzu.io
Pricing
Seat-based: This model charges based on the number of user seats or licenses allocated to an organization's individuals. Each seat typically corresponds to a specific user who can access the software, regardless of how often they use it.

Key features:
- Flexibility on data model changes: You can adjust the data model or add new events without impacting the performance.
- Collaboration features: You can easily embed your dashboards and charts to Notion or Craft for sharing.
- Quick customer support: Fast and efficient assistance whenever issues arise, ensuring minimal downtime.
- Super fast first integration: The setup and integration usually take less than an hour.
- Scalable architecture: Mitzu.io grows with your data volume, supporting terabytes to petabytes without performance degradation. Its pricing model is based on user seats rather than data volume, offering predictable costs as businesses scale.
- Advanced features: The platform supports retention analysis, funnel analysis, cohort segmentation, advanced segmentation, and subscription analytics (e.g., Monthly Recurring Revenue), making it particularly suitable for SaaS companies.
- Self-service BI: It reduces the reliance on data teams as non-technical team members are able to create insights.
- Data governance: 100% data reliability by syncing directly with the warehouse at every step, preserving compliance and security protocols. Also it eliminates data silos and duplication
Ideal use case
Mitzu.io is ideal for Saas companies and mid-sized organizations with high volume of data that are looking to democratize analytics across teams without requiring extensive technical expertise.
NetSpring (now part of Optimizely)
Pricing
It is not displayed by default; it is available upon request.

Key features
- Experimentation integration: It enables A/B testing directly on metrics stored in the warehouse. This helps businesses to measure the impact of experiments on real business outcomes without moving data out of the warehouse
- Unified event modeling: The platform consumes event streams in their native form (e.g., Salesforce entities like accounts or Zendesk tickets), providing rich business context for analytics.
- Self-service analytics: Users can leverage pre-built templates for attribution modeling, retention tracking, and more.
- User-friendly interface: Supports complex analytic computations through a UI-driven interface.
- Scalability: NetSpring’s architecture supports large-scale datasets and complex computations without compromising speed or accuracy.
- Flexibility in experimentation: It helps businesses to test variations across customer journeys while tying results directly to warehouse-stored metrics.
- Governance framework: Ensures consistent insights by maintaining the warehouse as the single source of truth.
Ideal use case
NetSpring (Optimizely) is best suited for organizations conducting large-scale experiments or managing complex datasets that require cross-functional insights.
Houseware (now part of LaunchDarkly)
Pricing
MTU-based: MTU-based pricing charges organizations based on the number of unique users actively engaging with the product within a given month.

Key Features
- No-code interface: Houseware enables users to build dashboards, funnels, retention analyses, and flows without writing SQL queries. This democratizes access to analytics across teams.
- Adaptive data support: Supports evolving data sources within modern architectures, ensuring compatibility across diverse datasets.
- Scalable architecture: Houseware’s architecture handles billions of rows of event-stream data efficiently while minimizing latency through intelligent caching strategies at multiple levels (browser, application, warehouse).
- Cost efficiency: By eliminating SDKs or external tools, Houseware reduces operational costs while maintaining high performance.
- Data privacy & control: The platform ensures complete control over enterprise data by operating entirely within the customer’s secure warehouse environment.
- Semantic layer integration: Enables universal metric definitions across the organization, ensuring consistency in reporting.
- Customizable workspaces: These offer pre-built templates for rapid deployment of analytics workflows.
Ideal use case
Houseware is ideal for organizations seeking cost-effective scalability in their product analytics operations. Its no-code interface makes it particularly appealing for teams without dedicated technical resources.
Kubit
Pricing
MTU-based: MTU-based pricing charges organizations based on the number of unique users actively engaging with the product within a given month.

Key Features
- Dynamic configuration with exploratory insights: It supports virtual events, virtual properties, dynamic functions, and unstructured data analysis (e.g., JSON), enabling hypothesis testing before committing to lengthy engineering work.
- Self-service data modeling: It allows users to create dimension tables, custom join conditions, and schema changes without engineering input.
- Customer journey analytics: Tracks user behavior from acquisition through retention using time-series analysis and funnel conversion metrics.
- Real-time data access via delta sharing protocols: Kubit leverages secure real-time protocols like Delta Sharing to query massive amounts of product data directly from the warehouse without duplication or compute cost concerns.
- Zero engineering overhead: Kubit eliminates the need for SDKs or reverse ETL jobs by dynamically querying raw operational tables alongside clickstream/behavioral events, all inside the customer’s warehouse.
- Iterative data model improvements: Due to dynamic joins functionality, new attributes or dimensions added to schemas automatically apply retroactively without requiring backfills.
Ideal use case:
Kubit is best suited for mid-to-large enterprises seeking transparency in their analytics workflows while avoiding vendor lock-in. Its ability to analyze raw operational data alongside structured schemas makes it ideal for businesses aiming to optimize customer journeys at scale.
Conclusion
The rise of warehouse-native analytics platforms marks a significant shift in how organizations leverage their data for actionable insights. By operating directly on top of data warehouses like Snowflake, BigQuery, Clickhouse and Databricks, these platforms eliminate the inefficiencies of reverse ETL processes, reduce latency, and ensure robust data governance.
The decision to adopt a warehouse-native analytics platform ultimately depends on an organization’s priorities - whether it’s democratizing access to data, enabling experimentation, driving engagement, achieving scalability, or optimizing customer journeys.
In the era of modern data stacks, warehouse-native analytics is no longer just a trend - it’s an essential strategy for organizations aiming to stay competitive in a data-driven world.